What is Big Data
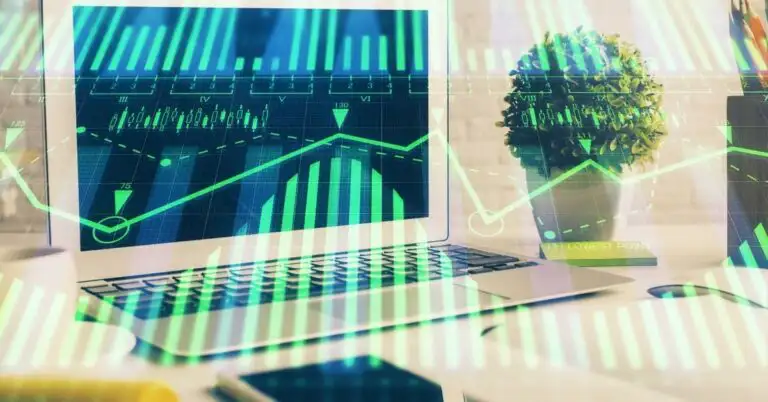
¿What is Big Data?
To begin explaining the concept of big data, it’s essential to start with the term ‘data,’ which essentially refers to data composed of metrics and dimensions.
Metrics are the quantities, symbols, characters, and numbers found in the data.
Dimensions, on the other hand, are attributes of the data. For example, the dimension ‘days of the week’ indicates the name of one or several days, such as ‘Tuesday, Thursday, etc.’
Types of Data
- Structured Data: This is data that can be organized in a fixed format, fitting into different columns of information, always conforming to this format, but whose content is composed only of text.
- Unstructured Data: These are data not necessarily classified in any apparent order and depend on search methods for their discovery. This category includes text, web pages, videos, images, etc., located on any server. For example, Google, as a search engine, has unstructured big data, which selects and classifies information based on the search.
- Semi-structured Data: Contains a mix of the two previous types of Big Data.
Examples of Big Data
Here are some examples of Big Data:
- The New York Stock Exchange: An example of Big Data that generates around one terabyte of new trading data per day.
- Social Networks: Statistics show that over 500 terabytes of new data are introduced into Facebook’s databases each day. This data is mainly generated in terms of photo and video uploads, message exchanges, comments, etc.
- A single jet engine can generate more than 10 terabytes of data in 30 minutes of flight time. With thousands of flights per day, data generation reaches many petabytes.
- Weather Prediction: Another example of Big Data. It requires collecting and analyzing vast amounts of data from various sources, including satellites, weather stations, and ocean buoys, to create accurate and useful weather prediction models.
Characteristics of Big Data
Big Data can be described by the following characteristics:
1. Volume
The name ‘Big Data’ itself relates to a size that is enormous. The size of the data plays a very important role in determining the data’s value. Whether a particular data set can be considered Big Data depends on the volume of data. Thus, ‘Volume’ is a characteristic that must be considered when dealing with Big Data solutions.
2. Variety
The next aspect of Big Data is its variety. Variety refers to heterogeneous sources and the nature of the data, both structured and unstructured. In the past, spreadsheets and databases were the only data sources considered by most applications. Nowadays, data in the form of emails, photos, videos, monitoring devices, PDF files, audio, etc., are also considered in analytic applications. This variety of unstructured data poses certain challenges for storage, mining, and data analysis.
3. Velocity
The term ‘velocity’ refers to the speed of data generation. The rate at which information is generated and processed to meet demands determines the real potential of the data. The velocity of Big Data refers to the speed at which data flows from sources like business processes, application logs, networks, social media sites, sensors, mobile devices, etc. The flow of data is massive and continuous.
4. Variability
This refers to the inconsistency that the data may show at times, making the process of managing and handling the data effectively more challenging.
Advantages of Big Data Processing
The ability to process Big Data in a DBMS (Database Management System) offers multiple benefits, such as:
1. Utilizing External Intelligence in Decision-Making
Access to social data from search engines and sites like Facebook and Twitter is enabling organizations to adjust their business strategies. This is one of the primary advantages of social media.
2. Improved Customer Service
Traditional customer feedback systems are being replaced by new systems designed with Big Data technologies. In these new systems, natural language processing and Big Data technologies are used to read and evaluate consumer responses, allowing for early identification of risks to products/services, if any.
3. Enhanced Operational Efficiency
Big Data technologies can be used to create a staging or landing area for new data before identifying which data should be moved to the data warehouse. Additionally, such integration of Big Data technologies and data warehousing helps an organization to offload infrequently accessed data.
Having the ability to visualize data and analyze Big Data today presents many benefits for companies. They can use it as input for strategic decision-making, such as adapting specific business actions based on data from Facebook and Twitter.
It can also enhance user experience, identify early the success or failure of a product, improve efficiency in business operations, and in the development of digital marketing strategies.
The Importance of Big Data in Marketing
The importance of Big Data in marketing doesn’t automatically translate to an improvement in marketing strategy, but the potential is there. Think of Big Data as your secret ingredient, your raw material, your essential element. It’s not the data itself that’s important, but the insights gained from Big Data, the decisions made, and the actions carried out.
By combining Big Data with an integrated marketing management strategy, marketing organizations can have a substantial impact in these key areas:
1. Customer Engagement
Big Data can provide insights not only about who your customers are, but also where they are, what they want, how they wish to be contacted, and when.
2. Customer Retention and Loyalty
Big Data can help you discover what influences customer loyalty and what keeps them returning repeatedly.
3. Marketing Optimization
With Big Data, you can determine the optimal marketing expenditure across multiple channels, as well as continuously optimize marketing programs through testing, measurement, and analysis.
Three Types of Big Data Important for Marketing
1. Customer Data
The most familiar category of big data for marketing may include behavioral, attitudinal, and transactional metrics from sources such as marketing campaigns, point of sale, websites, customer surveys, social media, online communities, and loyalty programs.
2. Operational Data
This category of big data typically includes objective metrics measuring the quality of marketing-related processes, including marketing operations, resource allocation, asset management, budget controls, etc.
3. Financial Data
Typically hosted in an organization’s financial systems, this category of big data may include sales, revenue, profits, and other types of objective data measuring the financial health of the organization.
Challenges of Big Data in Marketing
The challenges associated with the effective use of big data can be particularly daunting for marketing. This is because most analytical systems are not aligned with the data, processes, and decisions of the marketing organization. For marketing, three of the biggest challenges are:
1. Knowing What Data to Collect
Data is everywhere. You’re dealing with huge volumes of customer, operational, and financial data. But more isn’t always better: it has to be the right data.
2. Knowing Which Analytical Tools to Use
As the volume of big data grows, the available time to make decisions and act on them decreases. Analytical tools can help you aggregate and analyze data, as well as appropriately assign relevant information and decisions throughout the organization, but which ones?
3. Knowing How to Move from Data to Useful Information and then to Impact
Once you have the data, how do you convert it into useful information? And how do you use that information to have a positive impact on your marketing programs?
Steps from Big Data to Better Marketing
Big data is very important in marketing. But there are a few things every marketer should keep in mind to ensure that big data leads to great success:
1. Use Big Data for Deeper Understanding
Big data gives you the opportunity to delve deeper and deeper into the data, breaking it down to reveal richer insights. The insights gained from your initial analysis can be further explored, with richer, deeper insights emerging each time. This level of understanding can help you develop specific strategies and actions to drive growth.
2. Provide Big Data Insights to Those Who Can Use Them
CMOs need the meaningful insights that big data can provide; but so do store managers, call center staff, sales associates, and so on. What good is an insight if it remains within the confines of the boardroom? Make sure it reaches the hands of those who can act on it.
3. Don’t Try to Save the World
Tackling big data can sometimes feel overwhelming, so start by focusing on a few key goals. What outcomes would you like to improve? Once you decide that, you can identify which data would support the related analysis. After completing that exercise, move on to the next goal. And then the next.
4. Hire Experts
As companies collect and store large amounts of data, it’s necessary to have big data experts who can analyze it and extract useful insights for the business. Raw data is useless if it cannot be analyzed and converted into useful information, and data science and advanced analytics are necessary for this task.
Furthermore, the field of big data is constantly evolving, and experts in this area are trained to keep up with changes and adapt to new technologies and tools. They can also help businesses identify the most relevant and applicable data sets for their specific marketing needs.
Big data experts can also help address challenges that arise when working with large data sets, such as data quality issues, privacy and security concerns, and the complexity of integrating data from different sources.
Juan Esteban Yepes